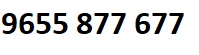

Python final year projects for cse in coimbatore
Looking for Best Python final year projects for cse in coimbatore ,then Intellimindz Technologies is the Best Final Year Project Center for cse in Coimbatore. We are also one of the popular Final year Project Center for cse in Coimbatore for Final Year Students. We are very genuine and caring about the Students project as well as their career development. Our project guides are from MNCs and Certified Experts having strong work Industry experience. We are the top rated Final year Project Center for cse in Coimbatore and we have unique style of guiding a student on the cse final year project.Academic project is not just another requirements in your degrees but also a chance to prove your talent to the industry. Intellimindz Technologies is the Best Final Year Project Center for cse in Coimbatore and we give a strong technical support for our students to get the projects done at the right time with the strong technical knowledge.Four years of college life would have thought you are lot of bookish knowledge and to transform your bookish knowledge into an industry standard project through the academic projects. The academic project that you work on shows the interest you have on the technologies, the skill level, but it will not happen until you get your hand a bit dirty today. The time you spend for the project is one that will make you successful in your job hunt.The final year project doesn’t just give you the hand in learning the real technology usage to make you fit for the industry but also groom you on team work,coordination, timely deadlines, and a lot of interpersonal development which will be required in the industry. Intellimindz Technologies is the ultimate destination of the students to have a successful Python final year projects for cse in coimbatore.
Python final year projects for cse in coimbatore
1. A Comprehensive Study on Social Network Mental Disorders Detection via Online Social Media Mining (Machine learning)
- The explosive growth in quality of social networking ends up in the problematic usage. associate degreeincreasing range of social network mental disorders (SNMDs), like Cyber-Relationship Addiction, data Overload, and internet Compulsion, are recently noted. Symptoms of those mental disorders ar typically ascertainedpassively nowadays, leading to delayed clinical intervention. during this paper, we have a tendency to argue that mining on-line social behavior provides a chance to actively determine SNMDs at associate degree early stage. it'sdifficult to discover SNMDs as a result of the mental standing can't be directly ascertained from on-line group action logs. Our approach, new and innovative to the apply of SNMD detection, doesn't admit self-revealing of these mental factors via questionnaires in science. Instead, we have a tendency to propose a machine learning framework, namely, Social Network disorder Detection (SNMDD), that exploits options extracted from social network knowledge to accurately determine potential cases of SNMDs. we have a tendency to conjointly exploit multi-source learning in SNMDD and propose a brand new SNMD-based Tensor Model (STM) to boost the accuracy. to extend the quantifiability of remembering, we have a tendency to more improve the potency with performance guarantee. Our framework is evaluated via a user study with 3126 on-line social network users. we have a tendency to conduct a feature analysis, and conjointly apply SNMDD on large-scale datasets and analyze the characteristics of the 3 SNMD varieties. The results manifest that SNMDD is promising for characteristic on-line social network users with potential SNMDs.
- Research of social recommendation aims at exploiting social info to enhance the standard of a recommender system. It is more divided into 2 categories. express social recommendation assumes the existence of not solelythe users’ ratings on things, however conjointly the specific social connections between users. Implicit social recommendation assumes the supply of solely the ratings however not the social connections between users, and tries to infer implicit social connections between users with the goal to spice up recommendation accuracy. This paper proposes a unified framework that's applicable to each express and implicit social recommendation. we have a tendency to propose associate degree improvement framework to be told the degree of social correlation and rating prediction put together, thus these 2 tasks will reciprocally boost the performance of every alternative. what is more, a widely known challenge for implicit social recommendation is that it takes quadratic time to be told the strength of try wise connections. This paper more proposes many sensible tricks to cut back the complexness of our model to be linear to the determined ratings. The experiments show that the projected model, with solely 2 parameters, will considerably exceed the progressive solutions for each express and implicit social recommender systems.
- As a big business paradigm, several on-line info platforms have emerged to satisfy society’s wants for person-specific knowledge, wherever a service supplier collects {raw knowledge|data|information} from data contributors, then offers added knowledge services to knowledge shoppers. However, within the knowledge commerce layer, the information shoppers face a pressing downside, i.e., a way to verify whether or not the service supplier has in truth collected and processed data? what is more, {the knowledge|the info|the information} contributors square measure sometimes unwilling to reveal their sensitive personal data and real identities to the informationshoppers. during this paper, we tend to propose TPDM, that expeditiously integrates honestness and Privacy preservation in knowledge Markets. TPDM is structured internally in associate Encrypt-then-Sign fashion, mistreatment part homomorphic secret writing and identity-based signature. It at the same time facilitates batch verification, processing, and outcome verification, whereas maintaining identity preservation and knowledgeconfidentiality. we tend to conjointly instantiate TPDM with a profile matching service and an informationdistribution service, and extensively assess their performances on Yahoo! Music ratings dataset and 2009 RECS dataset, severally. Our analysis and analysis results reveal that TPDM achieves many fascinating properties, whereas acquisition low computation and communication overheads once supporting large-scale knowledgemarkets.
- Online reviews became a vital supply of data for users before creating associate informed purchase call. Early reviews of a product tend to own a high impact on the following product sales. during this paper, we tend to take the initiative to check the behavior characteristics of early reviewers through their announce reviews on 2 real-world giant e-commerce platforms, i.e., Amazon and Yelp. In specific, we tend to divide product life into 3consecutive stages, particularly early, majority and laggards. A user United Nations agency has announce a review within the early stage is taken into account as associate early reviewer. we tend to quantitatively characterize early reviewers supported their rating behaviors, the helpfulness scores received from others and also thecorrelation of their reviews with product quality. we've found that (1) associate early reviewer tends to assign the next average rating score; associated (2) an early reviewer tends to post a lot of useful reviews. Our analysis of product reviews additionally indicates that early reviewers’ ratings and their received helpfulness scores square measure probably to influence product quality. By viewing review posting method as a multiplayer competition game, we tend to propose a completely unique margin-based embedding model for early reviewer prediction. intensive experiments on 2 totally different e-commerce datasets have shown that our planned approach outperforms variety of competitive baselines.
- Frequent itemsets mining with differential privacy refers to the matter of mining all frequent itemsets whose supports ar higher than a given threshold in an exceedingly given transactional dataset, with the constraint that the strip-mined results mustn't break the privacy of any single dealing. Current solutions for this drawback cannot well balance potency, privacy, and information utility over large-scale information. Toward this finish, we have a tendency to propose associate economical, differential personal frequent itemsets mining formula over large-scale information. supported the concepts of sampling and dealing truncation victimization length constraints, our formula reduces the computation intensity, reduces mining sensitivity, and therefore improves information utility given a set privacy budget. Experimental results show that our formula achieves higher performance than previous approaches on multiple datasets.
- Personalized recommendation is crucial to assist users notice pertinent info. It usually depends on an oversizedassortment of user knowledge, above all users’ on-line activity (e.g., tagging/rating/checking-in) on social media, to mine user preference. However, cathartic such user activity knowledge makes users liable to logical thinkingattacks, as non-public knowledge (e.g., gender) will usually be inferred from the users’ activity knowledge. during this paper, we have a tendency to planned PrivRank, a customizable and continuous privacy-preserving social media knowledge business framework protective users against logical thinking attacks whereas sanctionativecustomized ranking-based recommendations. Its key plan is to endlessly change user activity knowledge such the privacy outflow of user-specified non-public knowledge is decreased beneath a given knowledge distortion budget, that bounds the ranking loss incurred from the info obfuscation method so as to preserve the utility of the info for sanctionative recommendations. Associate in Nursing empirical analysis on each artificial and real-world knowledgesets shows that our framework will expeditiously give effective and continuous protection of user-specified non-public data, whereas still protective the utility of the obfuscated knowledge for customized ranking-based recommendation. Compared to progressive approaches, PrivRank achieves each an improved privacy protection and the next utility altogether the ranking-based recommendation use cases we have a tendency totested.
2. A General Framework for Implicit and Explicit Social Recommendation (Machine learning)
3. Achieving Data Truthfulness and Privacy Preservation in Data Markets (Data Mining)
4. Characterizing and Predicting Early Reviewers for Effective Product Marketing on E-Commerce Websites (Artificial Intelligence)
5. Frequent Itemsets Mining With Differential Privacy Over Large-Scale Data (Data Mining)
6. Privacy-Preserving Social Media Data Publishing for Personalized Ranking-Based Recommendation (Machine learning)
More
Python final year projects for cse trainer Profile
Our Final year projects Trainers
- More than 10 Years of experience in Final year Projects
- Has worked on multiple realtime projects
- Working in a top MNC company in Coimbatore
- Trained 2000+ Students so far
- Strong Theoretical & Practical Knowledge
- certified Professionals
Python final year projects for cse Locations in Coimbatore
-
Are You Located In Any Of These Areas
100 Feet Road,Avinashi Road,Gandhipuram,Koundampalayam,Kovipudur,Peelamedu,Ram Nagar,Ramanathapuram,Vadavalli RS Puram,Sai baba Colony,Saravanampatti,Shivandhapuram,Singanallur,sulur,Tatabad,Thudiyalur,Town Hall,Upplilipalayam
Intellimindz Saravanampatti branch is just few kilometre away from your location. If you need the Best training in Coimbatore, driving a couple of extra kilometres is worth it!